AI for product managers: A high-level guide
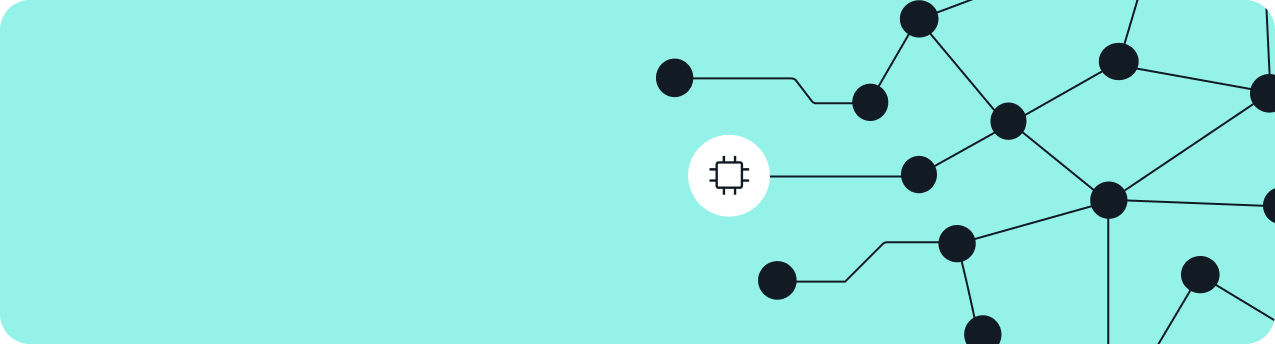
- Blog
- Augmented Apps
- Build Great Experiences
- Catch Up
- Embedded Analytics
Adding AI to your apps is becoming table stakes for builders. Dig into how to delight users with AI.
- How AI adds value to your product
- Getting started: Product managers must begin with the 'why'
- Identifying what AI functionality to add to your product
- Adding AI into your product: Build vs. buy
- To deliver better AI products, focus on your data
- Ensure your data science team is building a robust model
- K-fold cross-validation to resolve for model fit
- Building AI-powered products, boldly
Artificial intelligence (AI) holds immense significance for product managers because it empowers them to enhance their products in numerous ways, thus engaging customers and driving the success of their software or services. A recent Gartner survey on the usage of AI in organizations reports that 47% of respondents indicated that they are already using AI, and 64% plan to deploy new AI projects within the next two years.
By leveraging AI technologies, product managers can offer more personalized UX, predictive analytics, and conversational experiences, all of which improve customer satisfaction and retention. In increasingly competitive markets, AI-driven features such as natural language processing (NLP) and recommendation systems have the power to differentiate products, leading to increased customer engagement and loyalty.
Integrating AI for product managers means shipping products that include a multitude of AI/ML-driven innovations and ensuring that the product not only meets growing customer demand but also beats the competition. Ultimately, adding AI and ML to your product or service is crucial to ensure your product is competitive, and that its relevance to your most important audiences will only grow in the coming years.
How AI adds value to your product
Doing AI right means listening to your end-users, thinking deeply about how your customers will adopt these features within your product, and then matching those customer needs to the correct AI or ML technologies to incorporate.
AI can add value to your product or service in many ways, including:
- Elevating your product or service’s value proposition
- Increasing customer satisfaction
- Providing strong user engagement
- Reducing customer churn
- Opening up upsell opportunities for your product line
- Improving your customers’ business KPIs
In this article, we’ll share how product managers can build a plan to incorporate AI and machine learning into their products or services. We’ll also present key foundational considerations for you and your team to discuss.
Turn your data into immediate action: Unlocking end-to-end AI for analytics: From ML to GenAI makes analytics actionable.
Getting started: Product managers must begin with the ‘why’
Product managers considering adding AI to their products must take into account several key considerations. First, they should ensure that the integration of AI is driven by value and is user-centric. Don’t adopt AI just because it’s trending. Align its implementation with user needs and experiences. Second, assessing the technical feasibility of incorporating AI is crucial, it’s important to understand the capabilities and limitations of AI technologies before integration. Third, to guide the AI integration process effectively, product managers need to prioritize obtaining real-world insights and feedback. AI dashboards play a major role in this process, offering real-time visibility into metrics and system efficiency, enabling data-driven decisions that enhance AI adoption.
Suppose you’re a product manager in charge of an e-commerce application and are evaluating ways to incorporate AI/ML into your product. Talking to existing customers and getting feedback from prospective customer will help you understand specifically how AI might improve their experience and business outcomes.
One way to start learning about the “why” is by using an affinity diagram to prioritize and plan the minimum viable product (MVP). They are a useful tool for product managers when considering the integration of AI into a product.
How an affinity diagram can be used to integrate AI into a product:
- Gather ideas: Begin by conducting brainstorming sessions, workshops, or interviews with stakeholders. This may include users, customers, developers, and other relevant team members. Encourage them to share their ideas, concerns, and suggestions regarding the integration of AI into the product.
- Identify themes: Collect all the generated ideas and categorize them into meaningful themes or categories.
- Create and label affinity groups: After identifying the themes, create affinity groups by clustering similar ideas together. Each group will represent a cluster of ideas with significant similarities or associations.
- Analyze and prioritize: Review and analyze the different affinity groups. Identify which groups are most critical or are high priority for AI integration in the product. Consider feasibility, user value, technical requirements, and business objectives.
This approach is valuable for any product decision. However, it can be helpful to quickly—but completely—identify and organize insights and opportunities for AI integration, due to the market pressure to deliver that product managers are under.
Insights gathered can guide product managers in making informed decisions about how to add AI features to the product in a strategic way. The diagram serves as a visual aid for communicating the findings and recommendations to the team and stakeholders.
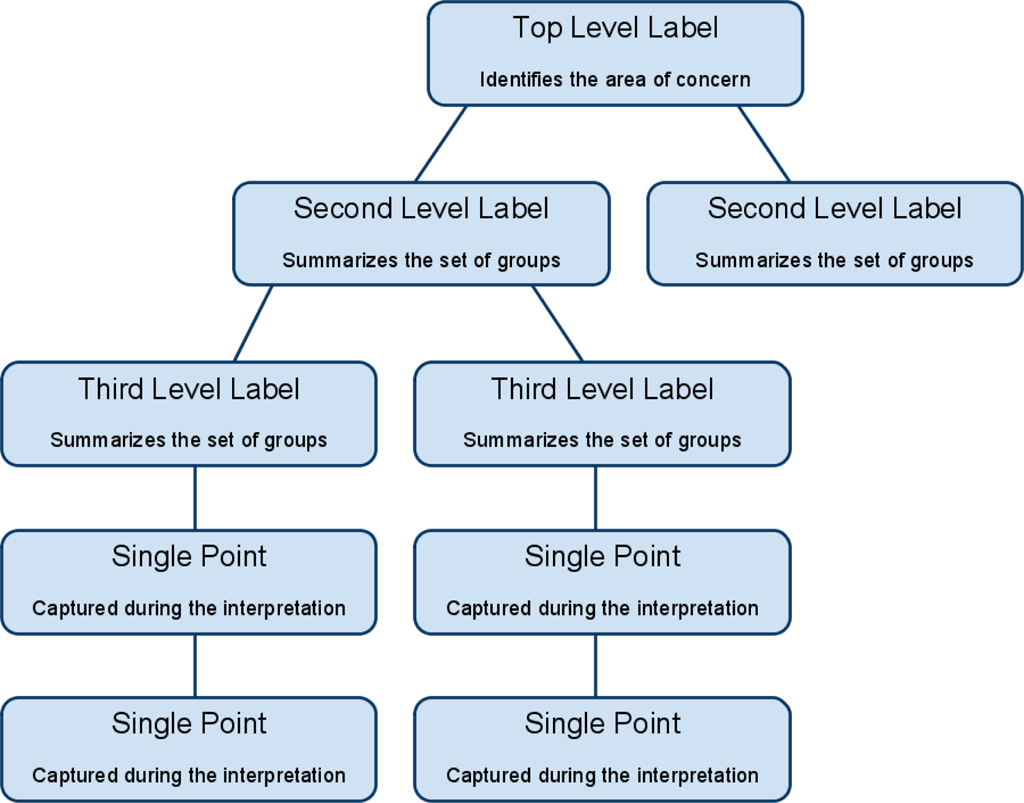
Identifying what AI functionality to add to your product
Let’s say as part of this exercise, you identify an opportunity to add a recommendation engine to your e-commerce product. The engineer is building for end-users who are e-commerce managers. So, the engineer provides analytics and recommendations regarding SKUs to stock. Based on customer buying patterns, the engineer also provides analytics on inventory management opportunities.
The next step is to drill into the business value and key functionality. Since you’re targeting e-commerce managers, our focus—based on market feedback—might be to include an AI-powered recommendation engine that can offer analytics and insights to e-commerce managers into which products are gaining popularity and which aren’t. The application can recommend the most relevant items to showcase on the e-commerce platform and help eliminate those not performing. With this information, e-commerce managers can make data-driven decisions regarding which products to stock in their warehouse and how to price them.
For inventory management, the AI recommendation engine can provide data on which products are in high demand, and also make recommendations on an inventory and restock plan. This information can help managers avoid inventory shortages and overstocks. E-commerce managers can use predictive analytics to ensure adequate inventory levels, prepare for seasonal demand, and optimize the supply chain.
Tips to help you identify what AI functionality to add to your product:
|
Adding AI into your product: Build vs. buy
Adding AI for product managers means making thoughtful decisions at several points in the product development process. Continuing our previous example, you’ve decided on our solution and functionality. At this point, you need to define how you’ll get to market. When product managers are adding AI analytics as well as embedded analytics to a product, they must make “build vs. buy” decisions based on several factors.
Building from the ground up might make sense if the organization has highly unique needs that cannot be met by existing AI and embedded analytics products in the market.
On the other hand, buying an embedded analytics solution that provides AI capabilities built-in can accelerate time to market. It’s also often more cost-effective to acquire an embedded analytics platform that already incorporates AI. Additionally, third-party embedded analytics platforms can enable teams to meet the full range of descriptive, predictive, and prescriptive needs, without requiring the vast amount of development and ongoing maintenance costs associated with building a solution or relying heavily on non-secure, hard-to-scale open-source options.
Product teams may also adopt a hybrid approach in which certain components are built in-house, and others are provided through an embedded analytics platform and generative AI.
To deliver better AI products, focus on your data
Having the right data (and enough of it) to train your AI is vital. You must ensure AI is well-trained on a robust data set that has been properly prepared. Accurately prepared data is the base of successful AI.
As a product manager responsible for providing AI, below are some important data-related questions your team should ask. The answers to these questions will help you and your team understand what type, and volume of data, is required to train your AI.
Questions to guide product managers and their teams to train the AI for their specific product:
|
High-quality data is essential for training robust AI models. Ensure that the data is accurate, complete, relevant, and consistent. Product teams must address issues such as missing values, outliers, duplicates, and errors before feeding the data into the AI algorithms.
In many cases, data engineering and building pipelines can be extremely code-intensive. Using data preparation tooling can help ensure:
- Correctness. Common data errors include Fahrenheit/Celsius, UTC, and a 12/24-hour clock conversion.
- Timeliness. Data must be relevant within the time scope, and not expired.
- Consistency. Validate consistent data types with test cases.
- Consolidation of data. Determine the output desired. For example, data for regression vs. classification should be distinguished by the ML model in use.
Turn your data into immediate action: Unlocking end-to-end AI for analytics: From ML to GenAI makes analytics actionable.
Ensure your data science team is building a robust model
As a product manager, you’ll want to ensure you get comfortable with the common issues and considerations of model development. Although AI product managers may not be involved at the level of algorithm development, they can benefit from recognizing the symptoms of overfitting in the behavior of the model under development.
When building your AI model, it’s vital to avoid both underfitting and overfitting. This is done by an ML method called validation. An underfitted model is inflexible and doesn’t learn well. An overfitted model learns from all the data, including noise in the data.
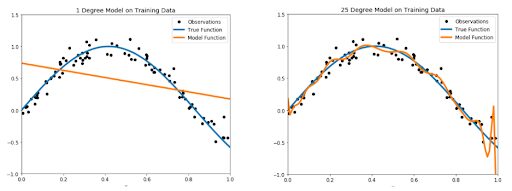
On the left, an underfitted model that seems to ignore the data. On the right, True Function more closely tracks the data.
The underfitted model looks as if it ignores the data altogether, while the overfitted model on the right shows even the noise in the data, which isn’t desirable. Without getting too technical, we’ll give you an example of validation that can be used to resolve these problems: k-fold cross-validation.
K-fold cross-validation to resolve for model fit
Here, you can see why it’s beneficial for AI product managers to have a data science background. The end goal is to deliver valuable functionality for your users. So, the ability to understand ML models at a high level improves a product manager’s aptitude for working directly with a team of data scientists to create accurate models.
K-fold cross-validation is useful because it helps avoid the problem of overfitting, where the model becomes too specialized to the training data and performs poorly on unseen data. It works by dividing the dataset into multiple subsets called “folds.” This method has the advantage of improving the model without requiring additional datasets. The model with the lowest cross-validation score will model the training data best while achieving a balance between underfitting and overfitting.
Sampling the mechanics of AI algorithms as we have just done leads us to the related topics of model selection and model evaluation. As a product manager, you’ll want to do your own research to learn about the best modeling methods for your AI product domain and scenario. Learning this kind of information will aid you in interacting meaningfully with your team of data scientists.
Building AI-powered products, boldly
To drive customer satisfaction and stay competitive, product managers need to add AI to their products. Whatever you’re building, data must be a part of it. Integrating AI and analytics enables product managers to deliver valuable, personalized, and intelligent solutions that not only meet user needs but also help their organization stay ahead in the market. Envision an AI-enhanced product that helps customers meet their goals, then build it boldly with the right data and AI.
Turn your data into immediate action: Unlocking end-to-end AI for analytics: From ML to GenAI makes analytics actionable.
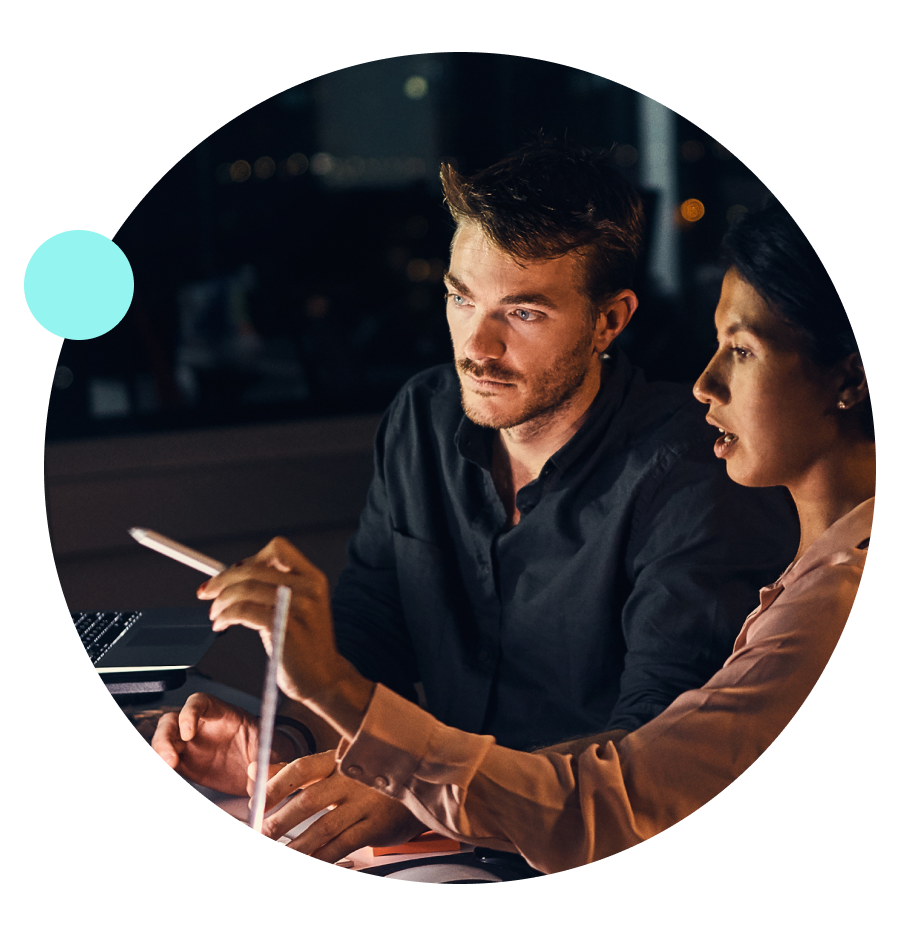