Building bridges: Data and BI teams partnering on an Analytics solution
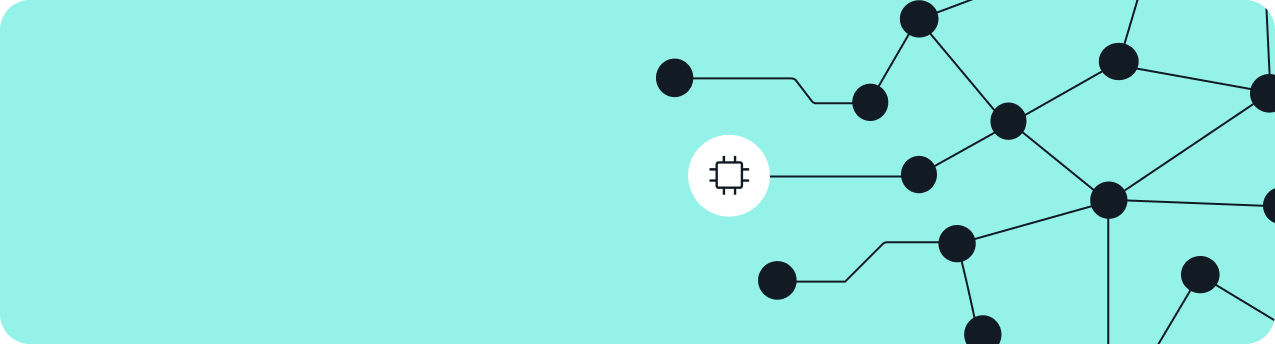
- Blog
- Building Bridges
- Fueling Data-Driven Businesses
- Unlock Complex Data
Implementing analytics at your company is a multi-team job. In Building Bridges, we focus on helping end users, app builders, and data experts select and roll out analytics platforms easily and efficiently. We live in a world driven by data….
Implementing analytics at your company is a multi-team job. In Building Bridges, we focus on helping end users, app builders, and data experts select and roll out analytics platforms easily and efficiently.
We live in a world driven by data. Access to information can be a game-changer for businesses looking to unlock strategic advantages through analytical insights. Ensuring that your organization has the right business intelligence and analytics tools to drive this innovation is key. As we have previously posted, the BI group is often the department that approaches the data teams for access to an analytics solution. But in this second post in our Building Bridges series, we are focused on the data teams driving the adoption of a BI and analytics tool with their business intelligence counterparts.
It’s no secret that data teams are becoming indispensable to organizations. Data is growing at an exponential rate and coming in from a variety of sources, making it more difficult to keep track of. This is one of the reasons we’ve seen the rise of data teams — they’ve grown beyond Silicon Valley startups and are finding homes in Fortune 500 companies. As data has become more massive, the technical skills needed to wrangle it have also increased. Big data is now modeled and queried using advanced coding languages like SQL, Python, and R. And rather than answering prescriptive questions — something that BI teams excel at — data teams are able to model future events and understand how changing a past variable could have affected the present.
So why do data teams reach out to their business intelligence colleagues to implement a new BI and analytics tool? The answer depends on the maturity of the organization and how the data team was formed.
Situation #1: Rapidly growing startup asking more business questions
The modern data team has gained traction in large part thanks to the startups in Silicon Valley that have put an emphasis on collecting, analyzing, and commoditizing data. These younger companies have invested in talent with specific data science skills, particularly with code-driven data analytics.
Data scientists, data engineers, and data analysts are hard to recruit and retain because they are in short supply. Furthermore, their scarcity means they command hefty salaries. Typically, a data team for a given startup ranges between two and five people. And as the business begins to grow, this team may not see additional investments.
At the same time, other business units in the company are now hiring BI analysts to help their department answer very specific questions. While the BI analysts have skills to ask questions of already modeled data, they often lack the coding acumen to query massive unstructured datasets in data lakes or cloud data warehouses. These BI analysts begin submitting requests to the data team, and as the business grows — and consequently the number of BI analysts increases — the small data team becomes overtaxed. Eventually, that data team, the bread-and-butter and differentiator for the startup, no longer can provide the same level of service for the business and executive team. Instead they must focus on a laundry list of queries from BI analysts throughout the organization.
This is the first use case of the data team needing to provide a business intelligence and analytics tool to the business. That tool must be flexible enough to allow the data team to query it with SQL, R, and Python, but must be accessible enough for the BI team to further engage with the data via visual graphical user interfaces and drill-down menus. By giving the BI analysts a self-service data dashboarding tool, the data team can get back to making major discoveries for the company.
Situation #2: Established company creates a data team for deeper insights
Today, established companies — Fortune 500 ones — have seen press around big data analytics and how the massive amount of information in their data warehouse can drive insights. They understand that there is power in data but realize that a different kind of skillset than their BI team has is needed to extract the value.
Often, an executive takes on the task of putting together a modern data team composed of professionals like data scientists, data engineers, and data analysts. They recruit some of the best and brightest, often away from startups, bringing them on to help drive insights. There’s one main problem: The tool that the BI team is using doesn’t allow the data team to query the information with code. At this point, the data team members may be thinking:
It is taking me too much time to answer the strategic questions that BI teams are asking because the tool that we’re using requires me to pre-model the data. Rather than using a solution driven by code, I’m getting behind because it’s taking me weeks to even answer a single question. The current tool is hamstringing my abilities, and I’m not able to use my full skillset.
This is a strong case for the data team to request a new BI and analytics tool to be used at their company. Clearly that team needs a place where they can write code and create visualizations of queries right away to quickly ascertain if the data is showing something that should be investigated further.
Implementing a tool that allows code, low-code, and no-code data visualization options
Regardless of the situation, the data team is well-served in building a bridge with their BI colleagues toward a solution that empowers the following:
1. Advanced code-driven data modeling: The solution should allow professionals the ability to model and visualize data by using the latest coding languages and techniques
2. Low-code data modeling: The solution should be accessible enough for business intelligence teams to join together different data models and tables to extract advanced insights
3. No-code data modeling and visualization: The solution should have the ability for nontechnical employees to model and view data dashboards specific to their industry and to drill down on the pieces of information that interest them the most
By having a solution, such as Sisense, that offers all three ways to interact with data, the organization will prevent the data team from performing redundant work of modeling data in one system and building models in another. This saves valuable time and effort for the smaller data team to tackle more advanced data modeling and answer the most strategic, higher-level questions of the organization.
Build a bridge to the BI team by bringing them into the process
As the data team looks to implement a new BI and analytics tool that can accommodate both their needs and those of their counterparts, it is crucial that the BI team is part of the process of selecting and testing the new tool. Having company-wide buy-in is crucial for adoption, and data teams are strongly encouraged to reach out to the BI team and build this bridge from the outset.
In particular, having the BI team part of the sales process from the beginning all the way to proof of concept is vital to make sure that everyone is on the same page. Clearly defining the roles of both the data and BI teams, coupled with having a comprehensive training program, will increase the odds of a successful adoption. The last thing any data team wants is to implement a new BI and analytics tool that is not adopted throughout the company. Data teams should reach across the organization to their BI teams to ensure collaboration throughout this process.
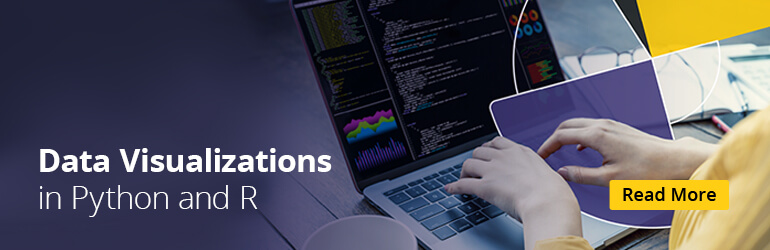
With over seven years of experience in a variety of technologies, former Sisenser Carmen DeCouto is dedicated to empowering advanced data teams as they tackle the next wave of industry-redefining challenges.
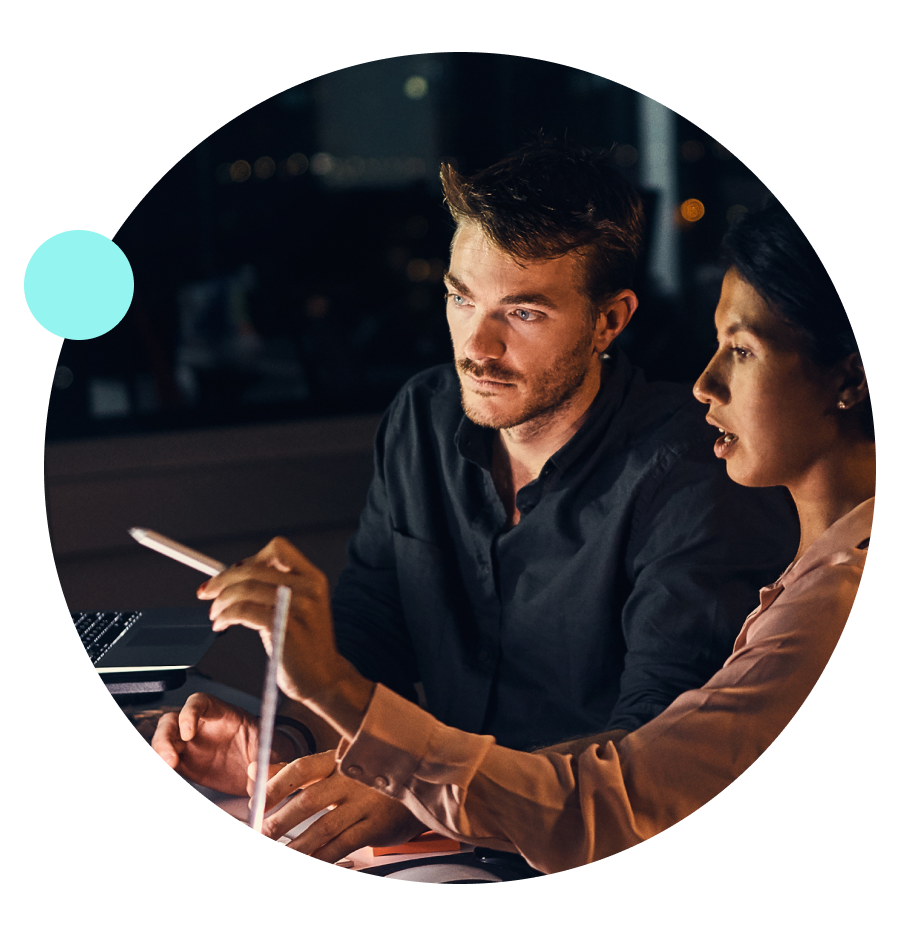