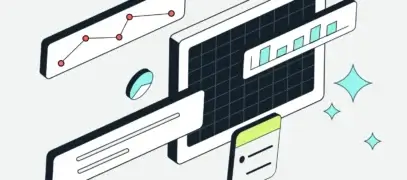
Sisense product roundup: Accelerating analytics with AI, semantic enrichment, and more in Q2 2025
This quarter’s updates help teams build tailored analytics faster with the launch of Sisense Intelligence and a wave of AI-powered tools. Explore new features for smarter dashboard design and enriched data models, to bring even more flexibility to your workflows.