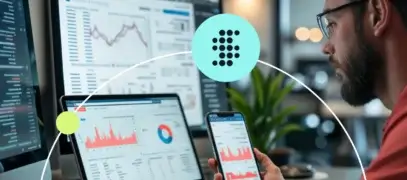
Supercharging developer productivity with Cursor, the AI code editor, and Sisense
Cursor is more than an AI helper; it’s a full-on coding partner embedded into your IDE. See how our team is using it inside Sisense to crush boilerplate, debug faster, and navigate legacy code like pros.