Predictive Data Analytics
What is Predictive Data Analytics?
Predictive data analytics uses current and past data to let you make predictions about the future or other unknowns. You can see the likelihood of a coming event or a specific situation, given the data being analyzed. Predictive data analytics examples are wide-ranging:
- Ecommerce sites use predictive analytics to offer specific products likely to interest a visitor. Predictions are based on that visitor’s past purchases and viewing of products.
See e-commerce analytics in action:
- A human resources department might use predictive analytics to detect if employees are thinking of quitting, and then persuade them to stay.
- In IT security, the prediction could be about where malware has infected systems, based on network activity and data flows. These systems then get top priority for in-depth inspection.
Predictive data analytics differs from general forecasting. It gives you insights into individual cases (individual customers, employees, and systems, in the examples above). This makes predictive analytics actionable. It opens the door to immediate improvements and results by applying the insights from the analytics.
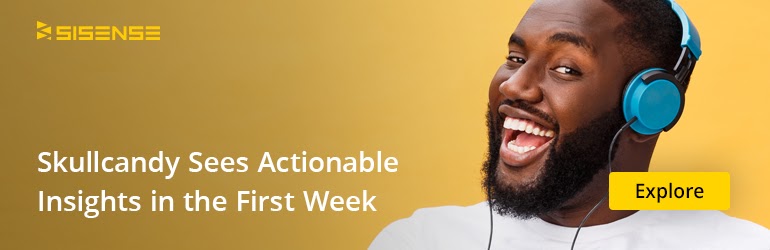
Basic steps for Predictive Data Analysis
Integrating the use of predictive data analytics can be done in the following steps:
- Define the result you want, e.g. how to offer each customer additional products of interest.
- Collect the data that will be needed (ecommerce site tracking data, CRM logs, etc.).
- As necessary, prepare the data from each source, then combine the different datasets.
- Make predictive analytics models, using statistical analysis to see which outcomes typically follow which events.
- Apply your models to your business.
- Review the models to ensure they are working properly.
User-friendly analytics software can make these steps accessible to business and non-technical users. You still need to decide which business benefit you want and identify the data required. After, the right software application can help make data preparation and combination simple, and the construction of predictive analytics models intuitive.
Bringing in Big Data
What is predictive analytics used for the most? It is especially useful when it comes to getting the most out of big data. The richness of big data can be leveraged for the highly specific insights per visitor. An example is the individual clicks on different products and pages of each visitor on an ecommerce site. Analytics techniques must then be adapted to high volume, velocity, and variety of data. One technique is data mining, which helps users identify patterns and trends. Others are statistical algorithms to build models, and machine learning to update models as new data arrives.
Keeping your data safe
Another area where predictive data analytics examples can help your organization raise the bar is cybersecurity. Using this method will go beyond where your systems have been attacked. It can predict where cybercriminals are likely to hit next, pinpoint your weak spots, and diagnose how prepared you are to withstand an attack before it happens.
Forecasting with Sisense
Using Sisense, you can easily change a parameter and see what it affects, so you can learn which scenario will help you achieve your goals and make the right business decisions. Sisense Forecast delivers highly sophisticated forecasting capabilities that turn business analysts into data heroes. Advanced machine learning algorithms can predict KPI outcomes from today’s data, all without Python scripting, R integration, or the use of commercial data science platforms.