5 Ways analytics is transforming retail and e-commerce
- Shifting to a unified view of the e-commerce business
- Achieving real-time reporting for proactive decision-making
- Empowering your retailer customers with self-service insight
- Aligning AI-powered analytics with a strong data foundation
- Driving next-level engagement through embedded analytics
- The future of embedded analytics in retail and e-commerce
- Conclusion: Embracing retail analytics techniques
For retail and e-commerce organizations, analytics are more important than ever. In fact, a recent retail analytics investment survey by Gartner reported, “In 2024, creating a strong foundation of analytics for unified retail commerce will help the business focus on fundamentals through, for example, more accurate forecasting, improved operational efficiencies and waste reduction for better sustainability.” That same survey reports that 87% of CIOs in the retail sector plan to increase their investment in analytics tools in 2024.
The insights gleaned from robust analytics solutions drive real-time decision-making. Improvements in operational efficiencies translate to a positive impact on customer engagement. However, despite the myriad benefits, there are common pitfalls that organizations need to avoid to maximize the effectiveness of their e-commerce analytics initiatives.
E-commerce organizations face a dizzying demand for insight across marketing, finance, customer service, and operations. Often the gap is filled with time-consuming spreadsheets, or making decisions based on gut instinct—which hurts business performance.
Organizations have a substantial opportunity to drive more engagement, broader visibility, and deeper analysis across every part of the e-commerce lifecycle, including:
- Website and marketing analytics. The best-run e-commerce companies have a clear lock on website and marketing performance and have shifted from spreadsheets to automated insight. They’ve moved away from conversion rates and understanding average order value and revenue generated from each marketing channel or campaign—and moved toward clear visibility into shopping cart abandonment and the drivers behind it.
- Product performance. These include valuable analytics on which products are most popular, identifying opportunities for cross-selling or upselling, optimizing pricing strategies, and analyzing product assortment and SKUs.
- Customer lifetime value. Insights into historical purchase data, average order value, repeat purchase rates, and customer retention rates enable e-commerce companies to target and focus on retaining the most profitable customers.
- Demand forecasting. Use the latest machine learning techniques to analyze historical sales, market trends, seasonality, and the impact of promotions to predict future demand. Demand forecasting also allows for the alignment of inventory and frictionless supplier relationships.
- Customer satisfaction analytics (CSAT). Track and analyze CSAT metrics, such as Net Promoter Score (NPS) or customer satisfaction ratings to manage customer loyalty, and identify trends and patterns. With this data, a team can proactively take action.
In this report, learn about traps to avoid, and be inspired by examples of leading retail and e-commerce organizations that harness analytics to streamline their operations, increase employee and end-user productivity, and make smart, data-driven decisions, and embed analytics in their daily user experiences.. Last, learn about the future of analytics in retail and e-commerce, from predictive inventory management to AI-powered experiences tailored to your most important audiences.
Shifting to a unified view of the e-commerce business
As an organization grows, so does its data, and so do the separate versions of the truth across the business. While that might work when an e-commerce business is small, growth often comes with the issue of data silos and fragmentation.
Data silos and fragmentation make it nearly impossible for teams to coordinate around decision-making and connect the dots across the organization. The problem is that data from, for example, sales transactions from the ERP, customer interactions from the CRM, and supply chain operations from the WMS may all be thrown into spreadsheets and databases without any consideration for how that data will reused, centralized, and shared across the business. And that inevitably leads to both redundant work and decisions based on old, incomplete, or just plain wrong data.
The fix: Robust data integration and strong data governance policies.
It’s vital to be able to access, organize, and analyze these troves of data coming in from different places. Why? Without proper integration and consolidation, disparate data can lead to incomplete or conflicting insights. To avoid the “disparate data” trap, look for robust data integration and strong data governance to be built into any analytics investment. This will pay off in the form of consistency and accuracy across all data sources, which enables leadership teams to meaningfully leverage that data in their most vital decision-making processes.
A single, unified view of data means that companies can drive stronger and more aligned performance across all aspects of e-commerce operations, including marketing, inventory, service desk, and more, by securely democratizing access to consistent, trusted data.
For instance, headphone manufacturer and industry leader Skullcandy struggled with siloed data sources. By partnering with Sisense, they achieved a single source of truth, allowing employees across departments to access and understand data independently. Brent Allen, Director of Infrastructure and WebOps at Skullcandy said, “Sisense allows data to be accessible in a way that everyone can see it, understand it, and filter it independently. That was probably the biggest win for us.” Indeed, the democratization of data empowers teams to make informed decisions quickly and accurately.
Sisense allows data to be accessible in a way that everyone can see it, understand it, and filter it independently. That was probably the biggest win for us.
– Brent Allen, Director of Infrastructure and WebOps, Skullcandy
In another example of data democratization success, Gousto, an online recipe box company, implemented Sisense Fusion Platform to enable real-time access to data for internal teams. Robert Barham, Director of Data at Gousto said, “Sisense can help us put data in the hands of users who in the past hadn’t been able to go as deep in our data as they want to be.”
Sisense can help us put data in the hands of users who in the past hadn’t been able to go as deep in our data as they want to be.
– Robert Barham, Director of Data, Gousto
Achieving real-time reporting for proactive decision-making
Real-time reporting and analytics are crucial for today’s e-commerce businesses. In an environment where quickly gauging the impact of a flash deal on revenue and profitability, or seeing how a rapidly emerging social media trend impacts sales demands and conversions, there is perhaps no other industry that is so tied to the need for timely decisions.
The huge shift from traditional, static reporting to having instant access to real-time insights cannot be underestimated. It allows e-commerce businesses to identify and respond to business and operating issues immediately. By quickly taking action, businesses are better able to leverage the opportunity in the moment.
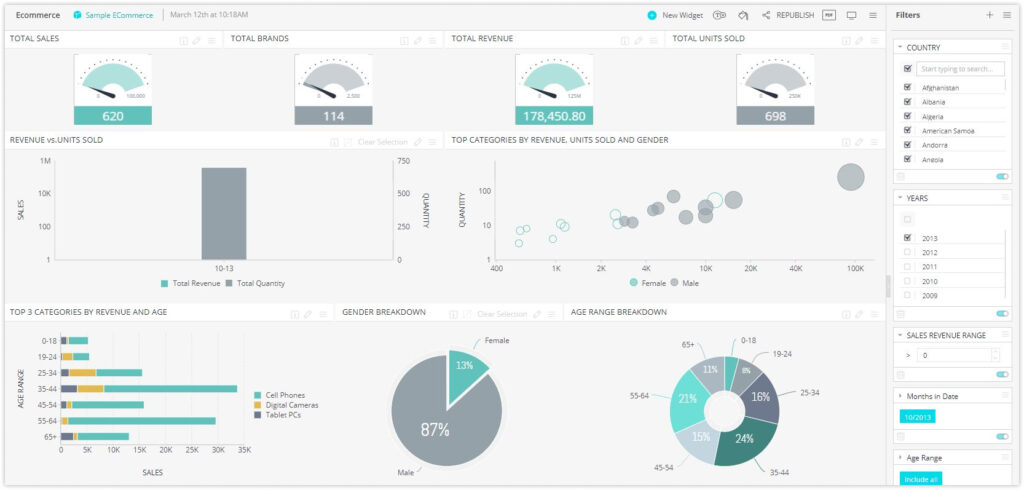
Use analytics to provide in-product reports and dashboards on sales trends, top-performing products, and more.
Empowering your retailer customers with self-service insight
So far, we’ve discussed critical considerations for applying analytics to e-commerce operations. But if you’re in the business of providing services to e-commerce companies and retailers, here’s a whole new opportunity: self-service insight. Whether you’re providing software and services to retailers and e-commerce companies for advertising, displays, product placement, or perhaps e-commerce technology from ERP to payment processing, the fact is your retailer customers are looking for you to provide better analytics as part of your service.
For instance, management consulting company Premium Retail Services (PRS), who provide services for proper product placement and effective displays to major retailers, wanted to transform their reporting capabilities for their customers. Rather than often having to manually create reports for their retail customer, they wanted to provide a complete self-service experience, so their customers could analyze store performance, compare with competitors, and evaluate different performances over different time ranges.
The results: Significant savings in labor costs for both their employees and customers, as well as increased customer satisfaction by empowering their clients to generate their own reports and manipulate data independently. PRS’ self-service approach fostered a culture of innovation and collaboration.
That said, beware of the trap of an overemphasis on tools over strategy. Yes, choose an analytics tool that empowers users and encourages the democratization of data. But avoid the pitfall of prioritizing technology over strategy. First and foremost, ensure a clear understanding of your team’s specific business and customer objectives and their analytical requirements.
The fix: Speak to your retail or e-commerce customers, learn what they are looking for, understand how much time your team is spending building reports for customers, and define your business objectives and analytical requirements accordingly.
Develop a comprehensive roadmap that outlines key priorities, resource allocation, and performance metrics. This approach ensures that your efforts deliver tangible value and drive business growth.
Aligning AI-powered analytics with a strong data foundation
Most e-commerce companies are operating their analytics without the benefits of the latest in AI, and missing out on some powerful opportunities. The latest machine learning (ML) algorithms can segment customers based on various attributes like demographics, behavior, and purchasing patterns to improve marketing performance. Machine learning can be used to increase forecast accuracy and shorten the cycle time to create a forecast. ML does so by analyzing historical sales data, product attributes, seasonality, and external factors to quickly build a robust forecast. For e-commerce companies with a vast array of SKUs, ML can be used to assist with price optimization so businesses can maximize revenue, maintain competitiveness, and improve profit margins. And that’s just the tip of the iceberg.
The problem organizations face is that it’s often hard to find AI/ML competency to build, or it’s too difficult for end users to understand. In addition, often the data to successfully drive ML requires data quality and enrichment improvements.
Platforms like Sisense provide AI/ML built-in and make it easy for users to get started. For example, if you want to provide demand forecasting analytics, your users can use Sisense’s built-in regression analysis to predict future demand based on historical sales data, taking into account seasonality or other relevant factors. Or, users can incorporate clustering to group customers based on their purchase history, demographic data, and other behavior.
However, a common pitfall is a lack of data quality and integrity. Inaccurate or incomplete data undermines the reliability of both traditional analytics and AI/ML-led projects alike. It can result in flawed analyses and misguided decision-making.
Organizations must prioritize data quality assurance processes, including data cleaning, validation, and enrichment. Algorithms are only as good as the data that drives them. These processes maintain the integrity of decisions and provide a powerful foundation to drive traditional and advanced analytics. Also: implement regular audits and monitoring mechanisms. Both will help to identify and rectify data inconsistencies sooner rather than later.
The fix: Regular audits and data monitoring. Ensure your analytics include data cleaning, validation, and enrichment.
Driving next-level engagement through embedded analytics
Whether you’re in the business of providing analytics to your retail customers or looking to drive increased engagement around analytics within your e-commerce team and business, embedded analytics is the enabler. It enables users to make decisions in the applications or user experiences they use every day, in context, without having to use separate analytics.
For example, let’s say you’re an e-commerce software provider who offers a platform for customers to run their e-commerce business. Using embedded analytics, you could provide in-product reports and dashboards on sales trends, top-performing products, and customer behavior so your customers could optimize marketing and merchandising. Or you could add embedded analytics to help your e-commerce customers better manage their inventory by providing real-time insights into inventory levels, stockouts, and excess inventory.
The key is to incorporate analytics into your users’ experiences so that it is natural, and they can take action at the point of decision. There are several ways to create more personalized analytics experiences and work on increasing engagement among your customers. Prioritize user-centric design principles when implementing embedded analytics solutions. Use composable development to integrate data visualizations and interactive analytics directly into your product that precisely match your look and feel, so it feels like a natural part of your overall product. Focus on intuitive interfaces, personalized dashboards, and user-friendly features so that your users can go from insight to action.
Invest in comprehensive training programs and change management initiatives, which can also help to encourage analytics adoption.
The fix: Embed analytics using composable development so that ad hoc analysis, visualizations, and recommendations, all feel like a natural part of your product or service.
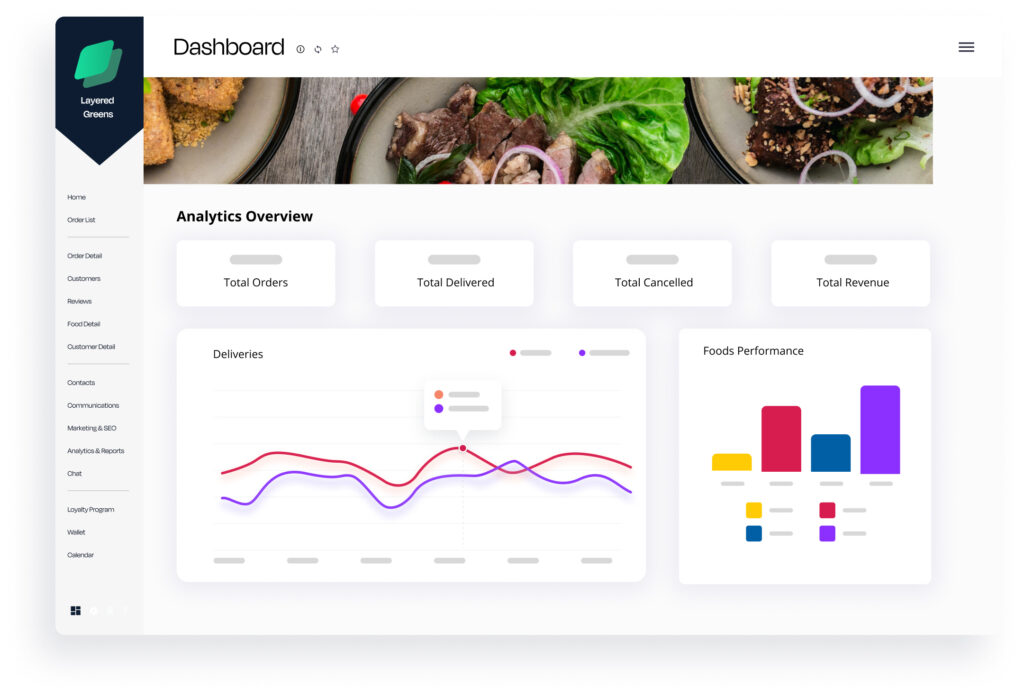
Embed analytics using composable development so that ad hoc analysis, visualizations, and recommendations, all feel like a natural part of your product or service.
For example, Luzern, a retail e-commerce technology company, leverages Sisense BloX to create visually appealing and actionable analytic apps for its customers. These apps provide real-time insights into marketplace sales performance, product placement, and competitor activities. The insights enable Luzern’s clients to make data-driven decisions, which in turn motivates their engagement and loyalty.
Patrick Sherlick, Chief Product Officer at Luzern said, “With Sisense BloX, my team has the functionality they need to build ‘full circle’ data experiences. BloX unleashes additional analytics functionality that will streamline workflows and decision-making in the e-commerce industry.”
With Sisense BloX, my team has the functionality they need to build ‘full circle’ data experiences. BloX unleashes additional analytics functionality that will streamline workflows and decision-making in the e-commerce industry.
– Patrick Sherlock, Chief Product Officer, Luzern
The future of embedded analytics in retail and e-commerce
Analytics in the retail and e-commerce sector holds immense opportunity. There are opportunities to revolutionize customer experiences, optimize operations, and drive growth. Read on for 5 ways that retail and e-commerce businesses can unlock new capabilities and redefine operations by using emerging analytics technologies.
Hyper-personalized customer experiences
By leveraging advanced machine learning algorithms and predictive analytics models, businesses can analyze vast amounts of customer data in real time to anticipate future purchasing decisions, recommend relevant products, and personalize marketing messages across multiple channels.
Even today, analytics enable retailers and e-commerce platforms to deliver hyper-personalized customer experiences tailored to individual preferences, behaviors, and needs. This level of personalization not only enhances customer satisfaction and loyalty but also drives higher conversion rates and revenue growth.
Predictive inventory management
Predictive retail analytics lets retailers optimize inventory management processes by accurately forecasting demand, identifying trends, and preventing stockouts or overstock situations. Real-time data from connected devices, such as RFID tags and sensors, enable retailers to track inventory levels, monitor product movements, and automate replenishment decisions. As a result, businesses can minimize carrying costs, reduce waste, and ensure seamless availability of products to meet customer demand.
AI-powered visual search and virtual try-on
Visual search and virtual try-on technologies powered by artificial intelligence (AI) and computer vision are already revolutionizing the way customers discover and interact with products online. By analyzing visual data, such as images and videos, AI algorithms can accurately identify products, recommend similar items, and even simulate virtual try-on experiences for apparel and accessories.
This immersive shopping experience not only enhances customer engagement but also reduces purchase hesitation and returns, driving higher conversion rates and customer satisfaction.
Augmented reality (AR) shopping experiences
AR technologies enable retailers to create immersive shopping experiences that blend the physical and digital worlds, allowing customers to visualize products in their real-world environments before making a purchase. By leveraging AR-powered mobile apps and smart devices, shoppers can virtually try out furniture, home decor, and cosmetics, experiencing products in context and making more informed buying decisions. This enhanced visualization capability not only drives engagement but also reduces uncertainty and boosts confidence in online purchases.
Ethical and sustainable analytics
In response to growing consumer awareness and demand for ethical and sustainable practices, analytics in the retail and e-commerce sectors will continue to prioritize transparency, traceability, and responsible data usage. Consumer demand will drive businesses to leverage analytics to track and measure the environmental and social impact of their operations, from sustainable sourcing and supply chain management to eco-friendly packaging and waste reduction initiatives.
By integrating ethical considerations into their embedded analytics strategies, retailers and e-commerce platforms can build trust with environmentally conscious consumers and differentiate themselves in the market.
Conclusion: Embracing retail analytics techniques
For the retail and e-commerce sectors, having the right analytics tools will drive innovation and competitive advantage. That said, organizations need to navigate specific traps to realize the full benefits. Address any challenges your organization has, including data silos, data quality, strategic alignment among business objectives and tools, and end-user adoption.
Embrace the analytics techniques and emerging technologies that align with your unique goals, and make a company-wide commitment to access to real-time insights, as well as ethical and sustainable analytics. Doing so means you’ll operate on new levels of innovation, efficiency, and customer-centricity.
To learn more about analytics solutions that will keep you competitive, schedule a demo.