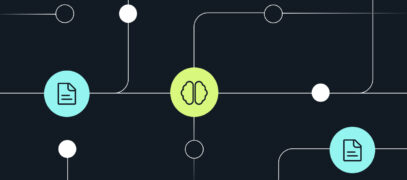
Unlocking end-to-end AI for analytics: From ML to GenAI
The AI-powered transformation of analytics Artificial Intelligence (AI) and Machine Learning (ML) have already brought about a seismic shift in the field of analytics, revolutionizing how data is processed, analyzed, and leveraged to drive business outcomes. For product teams, adding…